
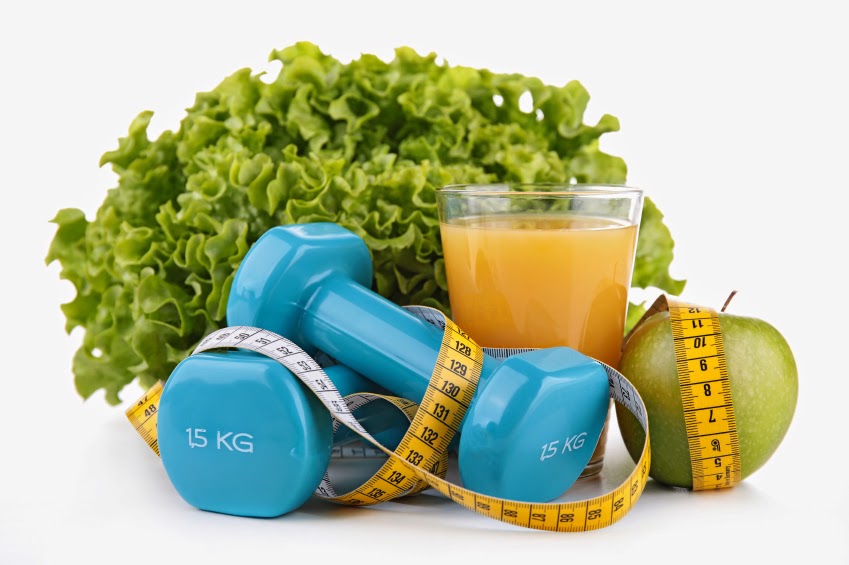
10.1016/j.cma.2013.04.003ĭormand, J R, Prince, P J: A family of embedded runge-kutta formulae. Center for Turbulence Research, Stanford University (2007)ĭoostan, A, Validi, A, Iaccarino, G: Non-intrusive low-rank separated approximation of high-dimensional stochastic models.

228 (12), 4332–4345 (2009)ĭoostan, A, Iaccarino, G, Etemadi, N: A least-squares approximation of high-dimensional uncertain systems. Journal of Guidance, Control, and Dynamics (2014)ĭoostan, A, Iaccarino, G: A least-squares approximation of partial differential equations with high-dimensional random inputs. 153, pp 1–14 (2014)ĭeMars, K J, Cheng, Y, Jah, M K: Collision probability with gaussian mixture orbit uncertainty. In: Proceedings of the 2nd IAA conference on dynamics and control of space system, Univelt San Diego, CA, vol. Journal of Guidance, Control, and Dynamics 37(2), 695–700 (2014)ĭell’Elce, L, Kerschen, G: Robust rendez-vous planning using the scenario approach and differential flatness. arXiv: 13050030 (2013)ĭeaconu, G, Louembet, C, Théron, A.: Minimizing the effects of navigation uncertainties on the spacecraft rendezvous precision. Courier Corporation (2013)Ĭhevreuil, M, Lebrun, R, Nouy, A, Rai, P: A least-squares method for sparse low rank approximation of multivariate functions. īrent, RP: Algorithms for minimization without derivatives. (2017)īeylkin, G, Garcke, J, Mohlenkamp, M J: Multivariate regression and machine learning with sums of separable functions. Celestial Mechanics and Dynamical Astronomy. AAS/AIAA Astrodynamics Specialist Conference Hilton Head, SC, August 11–15 (2013)īalducci, M, Jones B, Doostan A: Orbit uncertainty propagation and sensitivity analysis with separated representations.

AMS, Providence (1985)īalducci, M, Jones, B A, Doostan, A: Orbit uncertainty propagation with separated representations.
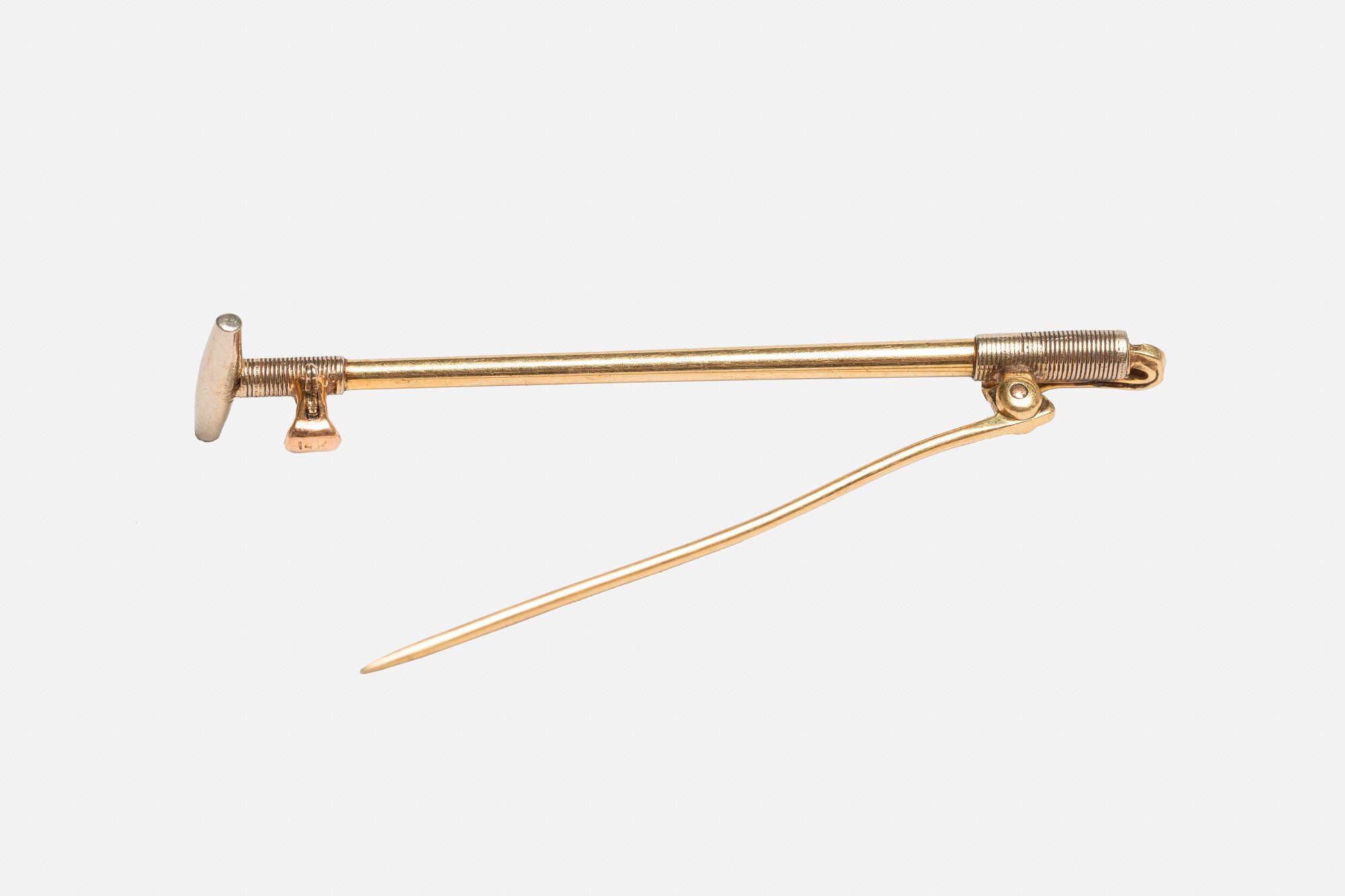
09–233 (2009)Īskey, R A, Arthur, W J: Some basic hypergeometric orthogonal polynomials that generalize Jacobi polynomials vol 319. AAS Spaceflight Mechanics Mtg, Pittsburgh, PA, Paper pp. The complete vector of input directions for the test case as well as the surrogateĪlfano, S: Satellite conjunction monte carlo analysis. Vector of deterministic factors for the separated representation Ξĭeterministic inputs to which the design inputs 𝜃 are mapped ξĪ vector of random input directions. Univariate functions in the separated representation u 0 The reliability index that represents the lower bound of an optimization under uncertainty constraint \(\bar \) u Polynomial coefficients for the separated representation χ Index for the collision in considering, i.e., 1 or 2 B Significance level of the confidence interval β Index indicating the considered object, i.e., 1, 2, or 3 α CI The accuracy of the surrogates is compared to that of a Monte Carlo reference, and the variability of the estimated probabilities of collision is analyzed. The probability of each collision is estimated via large numbers of samples propagated via the separated representation. The optimization problem therefore seeks to avoid both collisions. Two sequential, potential collisions are considered in the results of this paper, with one object able to maneuver. This efficiency is leveraged to improve tractability when solving the reliability design problem using optimization under uncertainty. Separated representations is a polynomial surrogate method that has been shown to be both efficient at propagating uncertainty when considering high stochastic dimension and accurate over long propagation times. This paper considers the methodology of separated representations for orbit uncertainty propagation and its subsequent application to a reliability design formulation of the maneuver design problem. When including constraints based on the probability of collision, any solution must be robust to the uncertainty of the system. Many current applications of maneuver design to astrodynamics consider a deterministic case, where statistics or uncertainty is left unquantified.
